FP-Recov
A simple and effective algorithm for extracting semantic information from floor plan images.
Abstract
As the acquisition of high-quality prior knowledge becomes easier, the use of prior
knowledge by robots is a focus of recent research. Among prior knowledge, architectural
floor plans are of great interest due to their availability and rich semantic information.
This paper proposes a novel neural network-based method for recovering semantic information from architectural floor plan images, combining convolutional neural networks
and graph neural networks to effectively improve the accuracy of the obtained semantic information. We conduct experiments on a challenging real-world floor plan image
dataset, and the results show that the proposed method can effectively extract semantic
information from floor plan images and generate high-quality vectorized results. The proposed method provides a new way for robots to use architectural floor plans, expanding
the range of prior information that robots can use.
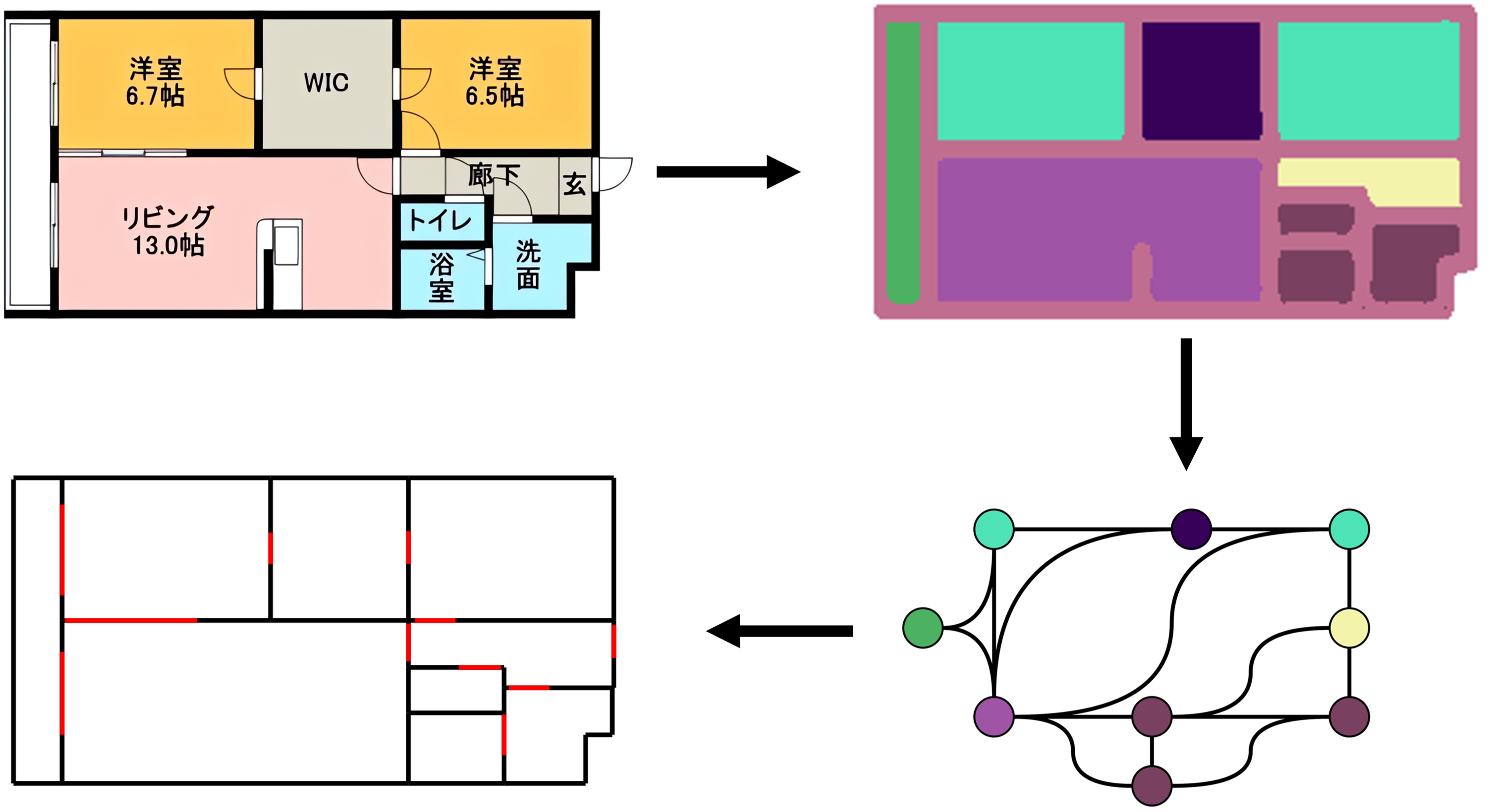
Code
Code borrows a lot from Raster-to-Vector: Revisiting Floorplan Transformation.